DFG (German Research Foundation) Project
Runtime: 36 Months
Partner: University of Bremen
We propose to combine Silent Speech Interfaces with Computational Paralinguistics to form Silent Paralinguistics (SP). To reach the envisioned project goal of inferring paralinguistic information from silently produced speech for natural spoken communication, we will investigate three major questions: (1) How well can speaker states and traits be predicted from EMG signals of silently produced speech, using the direct and indirect silent paralinguistics approach? (2) How to integrate the paralinguistic predictions into the Silent Speech Interface to generate appropriate acoustic speech from EMG signals (EMG-to-speech)? and (3) Does the resulting paralinguistically enriched acoustic speech signal improve the usability of spoken communication with regards to naturalness and user acceptance?
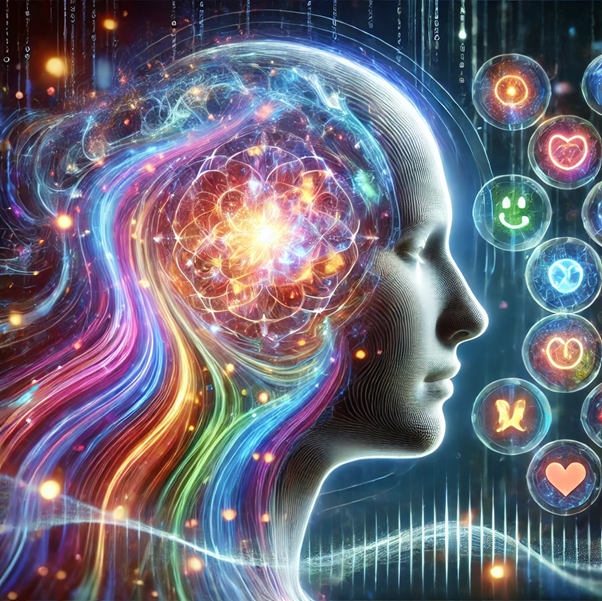
HearTheSpecies
Using computer audition to understand the drivers of soundscape composition, and to predict parasitation rates based on vocalisations of bird species (#SCHU2508/14-1)
(“Einsatz von Computer-Audition zur Erforschung der Auswirkungen von Landnutzung auf Klanglandschaften, sowie der Parasitierung anhand von Vogelstimmen“)
DFG (German Research Foundation) Project, Schwerpunktprogramm „Biodiversitäts-Exploratorien“
Runtime: 36 Months
Partner: University of Freiburg
The ongoing biodiversity crisis has endangered thousands of species around the world and its urgency is being increasingly acknowledged by several institutions – as signified, for example, by the upcoming UN Biodiversity Conference. Recently, biodiversity monitoring has also attracted the attention of the computer science community due to the potential of disciplines like machine learning (ML) to revolutionise biodiversity research by providing monitoring capabilities of unprecedented scale and detail. To that end, HearTheSpecies aims to exploit the potential of a heretofore underexplored data stream: audio. As land use is one of the main drivers of current biodiversity loss, understanding and monitoring the impact of land use on biodiversity is crucial to mitigate and halt the ongoing trend. This project aspires to bridge the gap between existing data and infrastructure in the Exploratories framework and state-of-the-art computer audition algorithms. The developed tools for coarse and fine scale sound source separation and species identification can be used to analyse the interaction among environmental variables, local and regional land-use, vegetation cover and the different soundscape components: biophony (biotic sounds), geophony (abiotic sounds) and anthropophony (human-related sounds).
SHIFT
SHIFT: MetamorphoSis of cultural Heritage Into augmented hypermedia assets For enhanced accessibiliTy and inclusion (#101060660)
EU Horizon 2020 Research & Innovation Action (RIA)
Runtime: 01.10.2022 – 30.09.2025
Partners: Software Imagination & Vision, Foundation for Research and Technology, Massive Dynamic, Audeering, University of Augsburg, Queen Mary University of London, Magyar Nemzeti Múzeum – Semmelweis Orvostörténeti Múzeum, The National Association of Public Librarians and Libraries in Romania, Staatliche Museen zu Berlin – Preußischer Kulturbesitz, The Balkan Museum Network, Initiative For Heritage Conservation, Eticas Research and Consulting, German Federation of the Blind and Partially Sighted.
The SHIFT project is strategically conceived to deliver a set of technological tools, loosely coupled that offers cultural heritage institutions the necessary impetus to stimulate growth, and embrace the latest innovations in artificial intelligence, machine learning, multi-modal data processing, digital content transformation methodologies, semantic representation, linguistic analysis of historical records, and the use of haptics interfaces to effectively and efficiently communicate new experiences to all citizens (including people with disabilities).
AUDI0NOMOUS
Agentenbasierte, interaktive, Tiefe 0-shot-learning-Netzwerke zur Optimierung von ontologischem Klangverständnis in Maschinen
# 442218748
DFG Reinhart Koselleck-Projekt
Soundscapes are a component of our everyday acoustic environment; we are always surrounded by sounds, we react to them, as well as creating them. While computer audition, the understanding of audio by machines, has primarily been driven through the analysis of speech, the understanding of soundscapes has received comparatively little attention.
AUDI0NOMOUS, a long-term project based on artificial intelligent systems, aims to achieve a major breakthroughs in analysis, categorisation, and understanding of real-life soundscapes. A novel approach, based around the development of four highly cooperative and interactive intelligent agents, is proposed herein to achieve this highly ambitious goal. Each agent will autonomously infer a deep and holistic comprehension of sound. A Curious Agent will collect unique data from web sources and social media; an Audio Decomposition Agent will decompose overlapped sounds; a Learning Agent will recognise an unlimited number of unlabelled sound; and, an Ontology Agent will translate the soundscapes into verbal ontologies.
AUDI0NOMOUS will open up an entirely new dimension of comprehensive audio understanding; such knowledge will have a high and broad impact in disciplines of both the sciences and humanities, promoting advancements in health care, robotics, and smart devices and cities, amongst many others.
Start date: 01.01.2021
Duration: 5 years
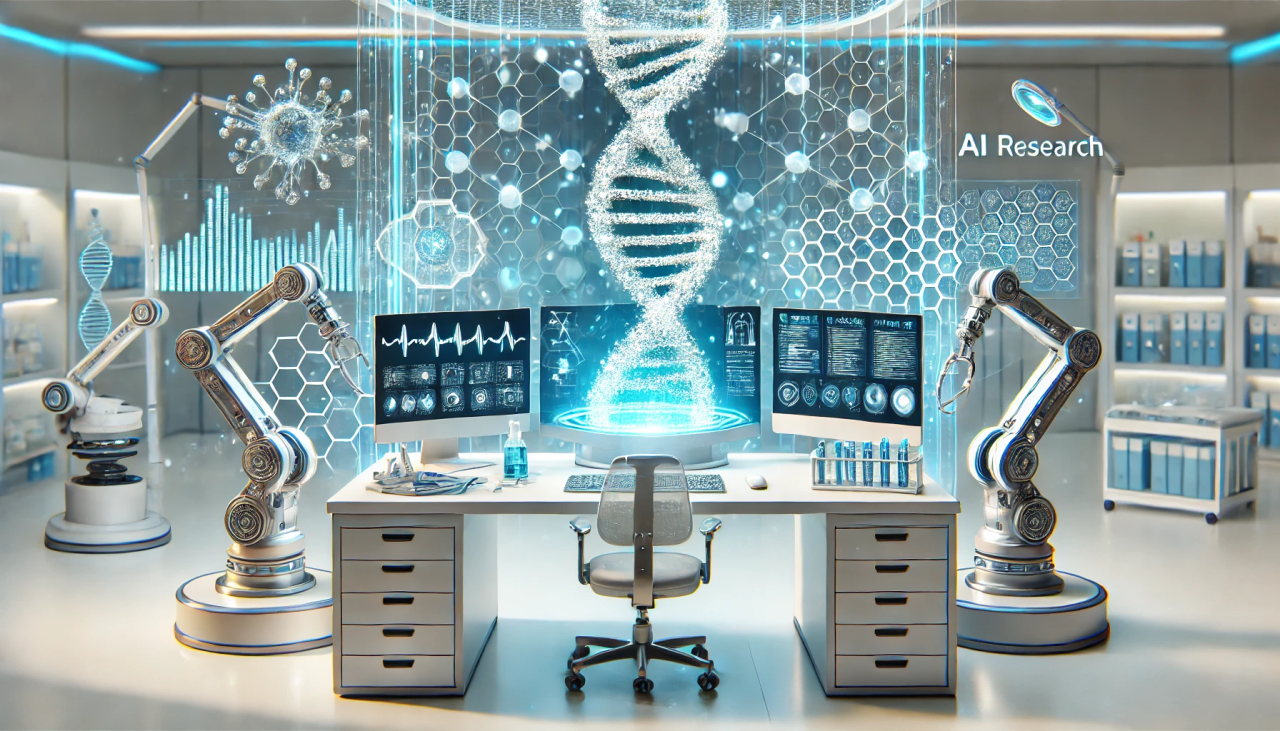
nature machine intelligence
Published: 07 February 2024(link is external)
Audio-based AI classifiers show no evidence of improved COVID-19 screening over simple symptoms checkers
Recent work has reported that respiratory audio-trained AI classifiers can accurately predict SARS-CoV-2 infection status. However, it has not yet been determined whether such model performance is driven by latent audio biomarkers with true causal links to SARS-CoV-2 infection or by confounding effects, such as recruitment bias, present in observational studies. Here we undertake a large-scale study of audio-based AI classifiers as part of the UK government’s pandemic response.
Science Direct
Published: 10 November 2023(link is external)
Zero-shot personalization of speech foundation models for depressed mood monitoring
Depression, as one of the most prevalent mental health diseases, negatively impacts millions of lives. Diagnoses are achieved by the assessment of symptoms with standardized tests. However, recent studies indicate that continuously monitoring symptoms (e.g., with ecological momentary assessments [EMAs]) may provide relevant additional information for both diagnosis and treatment decisions.
nature mental health
Published: 07 August 2023(link is external)
How to e-mental health: a guideline for researchers and practitioners using digital technology in the context of mental health
Despite an exponentially growing number of digital or e-mental health services, methodological guidelines for research and practical implementation are scarce. Here we aim to promote the methodological quality, evidence and long-term implementation of technical innovations in the healthcare system. This expert consensus is based on an iterative Delphi adapted process and provides an overview of the current state-of-the-art guidelines and practical recommendations on the most relevant topics in e-mental health assessment and intervention.
The Lancet
Published: 21 July 2021(link is external)
COVID-19 detection from audio: seven grains of salt
Digital mass testing for COVID-19 via a mobile phone application could be made possible through machine learning and its ability to identify patterns in data. COVID-19 appears to confer unique features in the audio produced by infected individuals and machine learning COVID-19 detection from breath, cough, and speech audio recordings has yielded promising results.